Life of Brian is an Ash Gaming-powered online slot featuring 5 reels and 30 paylines of winning opportunities. Inspired by the hilarious Monty Python stage show, this game will tickle the fancy of those looking to travel back in time to when the classic 1976 film was released. In addition to a unique theme, this game also features plenty of. Free Download - NEW SLOT! MONTY PYTHON & THE HOLY GRAIL KILLER BUNNY & BLACK KNIGHT. My Pechanga Slot Machine Videos. This is the Monty Python and the Holy Grail Slot Machine with the Killer Bunny and Black Night versions. I found these machines at Pechanga Resort and. Video Slots - Download & Play Online! The official online home for all things Monty Python. Pages of everything you'll ever need to know about Monty Python and their movies, TV shows, books, live stage shows, apps and latest projects, as well as exclusive videos, news and a Fanwall where all your #montypython content will live. Python Slot Machine Code; Python Slots Property Search; Python Slots Property Management; Monty Python Slot Machine App; Python Set Property; Class Property Python; Section author: e8johan. Last Build: December 07, 2020 at 10:50 CET. The source code for this chapter can be found in the assets folder. The datatype may be any Python type.
- Monty Python Holy Grail Free
- Holy Grail Slot Machine
- Monty Python Slots Online
- Monty Python Slot Machine App
Decorator for adding slots Python3.7 provides dataclasses module for faster class creation (PEP 557). Unfortunately there's no support for slots. Do it by yourself or use dataslots.dataslots decorator. Just for completeness of my notes, note that there is a one-time cost per slot in the class's namespace of 64 bytes in Python 2, and 72 bytes in Python 3, because slots. Just for completeness of my notes, note that there is a one-time cost per slot in the class's namespace of 64 bytes in Python 2, and 72 bytes in Python 3, because slots use data descriptors like properties, called 'members'. Foo.foo type(Foo.foo) class 'memberdescriptor' getsizeof(Foo.foo) 72.
slots provide a special mechanism to reduce the size of objects. It is especially useful if you need to allocate thousands of objects that would otherwise take lots of memory space. It is not very common but you may find it useful someday. Note, however, that it has some side effects (e.g. pickle may not work) and that Python 3 introduced memory optimisation on objects (sse http://www.python.org/dev/peps/pep-0412/) so slots may not be needed anymore ?
The main idea is as follows. As you may know every object in Python contains a dynamic dictionary that allows adding attributes. You can see the slots as the static version that does not allow additional attributes.
Contents
- slots
- Quick Example
Here is the slots syntax uing the __slot__ keyword:
The traditional version would be as follows:
This means that for every instance you'll have an instance of a dict. Now, for some people this might seem way too much space for just a couple of attributes.
Unfortunately there is a side effect to slots. They change the behavior of the objects that have slots in a way that can be abused by control freaks and static typing weenies. This is bad, because the control freaks should be abusing the metaclasses and the static typing weenies should be abusing decorators, since in Python, there should be only one obvious way of doing something.
Making CPython smart enough to handle saving space without __slots__ is a major undertaking, which is probably why it is not on the list of changes for P3k (yet).
I'd like to see some elaboration on the 'static typing'/decorator point, sans pejoratives. Quoting absent third parties is unhelpful. __slots__ doesn't address the same issues as static typing. For example, in C++, it is not the declaration of a member variable is being restricted, it is the assignment of an unintended type (and compiler enforced) to that variable. I'm not condoning the use of __slots__, just interested in the conversation. Thanks! – hiwaylon Nov 28 ‘11 at 17:541
Each python object has a __dict__ atttribute which is a dictionary containing all other attributes. e.g. when you type self.attr python is actually doing self.__dict__[‘attr']. As you can imagine using a dictionary to store attribute takes some extra space & time for accessing it.
However, when you use __slots__, any object created for that class won't have a __dict__ attribute. Instead, all attribute access is done directly via pointers.
So if want a C style structure rather than a full fledged class you can use __slots__ for compacting size of the objects & reducing attribute access time. A good example is a Point class containing attributes x & y. If you are going to have a lot of points, you can try using __slots__ in order to conserve some memory.
No, an instance of a class with __slots__ defined is not like a C-style structure. There is a class-level dictionary mapping attribute names to indexes, otherwise the following would not be possible: class A(object): __slots__= 'value',nna=A(); setattr(a, ‘value', 1) I really think this answer should be clarified (I can do that if you want). Also, I'm not certain that instance.__hidden_attributes[instance.__class__[attrname]] is faster than instance.__dict__[attrname]. – tzot Oct 15 ‘11 at 13:56up vote 4 down vote
Instant bingo games. Slots are very useful for library calls to eliminate the 'named method dispatch' when making function calls. This is mentioned in the SWIG documentation. For high performance libraries that want to reduce function overhead for commonly called functions using slots is much faster.
Now this may not be directly related to the OPs question. It is related more to building extensions than it does to using the slots syntax on an object. But it does help complete the picture for the usage of slots and some of the reasoning behind them.
By default, instances of both old and new-style classes have a dictionary for attribute storage. This wastes space for objects having very few instance variables. The space consumption can become acute when creating large numbers of instances.
The default can be overridden by defining __slots__ in a new-style class definition. The __slots__ declaration takes a sequence of instance variables and reserves just enough space in each instance to hold a value for each variable. Space is saved because __dict__ is not created for each instance.

This class variable can be assigned a string, iterable, or sequence of strings with variable names used by instances. If defined in a new-style class, __slots__ reserves space for the declared variables and prevents the automatic creation of __dict__ and __weakref__ for each instance. New in version 2.2.
Notes on using __slots__
Without a __dict__ variable, instances cannot be assigned new variables not listed in the __slots__ definition. Attempts to assign to an unlisted variable name raises AttributeError. If dynamic assignment of new variables is desired, then add ‘__dict__' to the sequence of strings in the __slots__ declaration. Changed in version 2.3: Previously, adding ‘__dict__' to the __slots__ declaration would not enable the assignment of new attributes not specifically listed in the sequence of instance variable names.
Without a __weakref__ variable for each instance, classes defining __slots__ do not support weak references to its instances. If weak reference support is needed, then add ‘__weakref__' to the sequence of strings in the __slots__ declaration. Changed in version 2.3: Previously, adding ‘__weakref__' to the __slots__ declaration would not enable support for weak references.
__slots__ are implemented at the class level by creating descriptors (3.4.2) for each variable name. As a result, class attributes cannot be used to set default values for instance variables defined by __slots__; otherwise, the class attribute would overwrite the descriptor assignment.
If a class defines a slot also defined in a base class, the instance variable defined by the base class slot is inaccessible (except by retrieving its descriptor directly from the base class). This renders the meaning of the program undefined. In the future, a check may be added to prevent this.

Warning
effects of a __slots__ declaration is limited to the class where it is defined. In other words, subclasses will have a __dict__ (unless they also define __slots__).
__slots__ do not work for classes derived from ``variable-length'' built-in types such as long, str and tuple.
Any non-string iterable may be assigned to __slots__. Mappings may also be used; however, in the future, special meaning may be assigned to the values corresponding to each key.
For every instance of any class, attributes are stored in a dictionary.
This means that for every instance you'll have an instance of a dict. Now, for some people this might seem way too much space for just a couple of attributes.
If you have lots and lots and looooots of instances, and you want to save some memory, you can use __slots__. The basic idea is that when you define the __slots__ class attribute, those attributes will get just the enough space, without wasting space.
Here is the previous example using __slots__:
Now, one side effect of these __slots__ thing is that, whenever you define the __slots__ class attribute, your __dict__ attribute for every instance will be gone!. It's not a surprise because that's why you should use __slots__ in the first place… to get rid off the __dict__ in every instance, to save some memory remember?Can't bind attributes to the instance any more…
Another side effect is that, as there is no __dict__, there is no way to add, at runtime, any attributes to your instance:
# This should should work if there is no __slots__ defined..>>> instance.new_attr = 10Traceback (most recent call last):
AttributeError: ‘myClass' object has no attribute ‘new_attr'>>>Read only attributes?
Another one is that, if there is some kind of collision between the slot and a class attribute, then the class attribute will overwrite the slot and, as there is no __dict__, the class attribute will be read-only.
However if you want to have a __dict__, you can always insert into the __slots__ the ‘__dict__' value, and all these little side effects will go away
But what if I wanted to add the ‘__dict__' value into __slots__ at runtime?
sorry dude but, no can do.
reference: http://mypythonnotes.wordpress.com/2008/09/04/__slots__/:wq
Latest versionReleased:
A multi-armed bandit library for Python
Monty Python Slot Machine App
Project description
A multi-armed bandit library for Python
Slots is intended to be a basic, very easy-to-use multi-armed bandit library for Python.
Author
Roy Keyes -- [email protected]
License: MIT
See LICENSE.txt
Introduction
Monty Python Holy Grail Free
slots is a Python library designed to allow the user to explore and use simple multi-armed bandit (MAB) strategies. The basic concept behind the multi-armed bandit problem is that you are faced with n choices (e.g. slot machines, medicines, or UI/UX designs), each of which results in a 'win' with some unknown probability. Multi-armed bandit strategies are designed to let you quickly determine which choice will yield the highest result over time, while reducing the number of tests (or arm pulls) needed to make this determination. Typically, MAB strategies attempt to strike a balance between 'exploration', testing different arms in order to find the best, and 'exploitation', using the best known choice. There are many variation of this problem, see here for more background.
slots provides a hopefully simple API to allow you to explore, test, and use these strategies. Basic usage looks like this:
Using slots to determine the best of 3 variations on a live website.
Make the first choice randomly, record responses, and input reward 2 was chosen. Run online trial (input most recent result) until test criteria is met.
The response of mab.online_trial()
is a dict of the form:
Where:
- If the criterion is met,
new_trial
=False
. choice
is the current choice of arm to try.best
is the current best estimate of the highest payout arm.
To test strategies on arms with pre-set probabilities:
To inspect the results and compare the estimated win probabilities versus the true win probabilities:
By default, slots uses the epsilon greedy strategy. Besides epsilon greedy, the softmax, upper confidence bound (UCB1), and Bayesian bandit strategies are also implemented.
Regret analysis
A common metric used to evaluate the relative success of a MAB strategy is 'regret'. This reflects that fraction of payouts (wins) that have been lost by using the sequence of pulls versus the currently best known arm. The current regret value can be calculated by calling the mab.regret()
method.
For example, the regret curves for several different MAB strategies can be generated as follows:
API documentation
For documentation on the slots API, see slots-docs.md.
Todo list
- More MAB strategies
- Argument to save regret values after each trial in an array.
- TESTS!
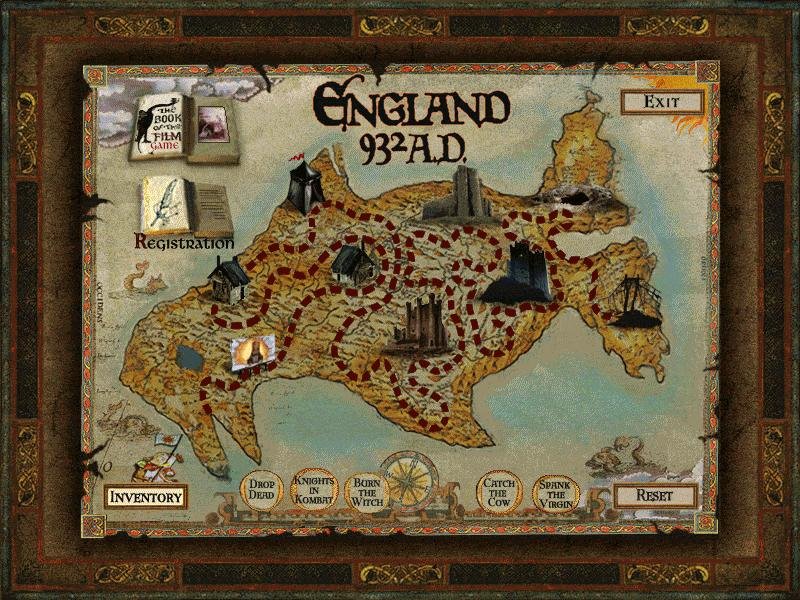
Contributing

This class variable can be assigned a string, iterable, or sequence of strings with variable names used by instances. If defined in a new-style class, __slots__ reserves space for the declared variables and prevents the automatic creation of __dict__ and __weakref__ for each instance. New in version 2.2.
Notes on using __slots__
Without a __dict__ variable, instances cannot be assigned new variables not listed in the __slots__ definition. Attempts to assign to an unlisted variable name raises AttributeError. If dynamic assignment of new variables is desired, then add ‘__dict__' to the sequence of strings in the __slots__ declaration. Changed in version 2.3: Previously, adding ‘__dict__' to the __slots__ declaration would not enable the assignment of new attributes not specifically listed in the sequence of instance variable names.
Without a __weakref__ variable for each instance, classes defining __slots__ do not support weak references to its instances. If weak reference support is needed, then add ‘__weakref__' to the sequence of strings in the __slots__ declaration. Changed in version 2.3: Previously, adding ‘__weakref__' to the __slots__ declaration would not enable support for weak references.
__slots__ are implemented at the class level by creating descriptors (3.4.2) for each variable name. As a result, class attributes cannot be used to set default values for instance variables defined by __slots__; otherwise, the class attribute would overwrite the descriptor assignment.
If a class defines a slot also defined in a base class, the instance variable defined by the base class slot is inaccessible (except by retrieving its descriptor directly from the base class). This renders the meaning of the program undefined. In the future, a check may be added to prevent this.
Warning
effects of a __slots__ declaration is limited to the class where it is defined. In other words, subclasses will have a __dict__ (unless they also define __slots__).
__slots__ do not work for classes derived from ``variable-length'' built-in types such as long, str and tuple.
Any non-string iterable may be assigned to __slots__. Mappings may also be used; however, in the future, special meaning may be assigned to the values corresponding to each key.
For every instance of any class, attributes are stored in a dictionary.
This means that for every instance you'll have an instance of a dict. Now, for some people this might seem way too much space for just a couple of attributes.
If you have lots and lots and looooots of instances, and you want to save some memory, you can use __slots__. The basic idea is that when you define the __slots__ class attribute, those attributes will get just the enough space, without wasting space.
Here is the previous example using __slots__:
Now, one side effect of these __slots__ thing is that, whenever you define the __slots__ class attribute, your __dict__ attribute for every instance will be gone!. It's not a surprise because that's why you should use __slots__ in the first place… to get rid off the __dict__ in every instance, to save some memory remember?Can't bind attributes to the instance any more…
Another side effect is that, as there is no __dict__, there is no way to add, at runtime, any attributes to your instance:
# This should should work if there is no __slots__ defined..>>> instance.new_attr = 10Traceback (most recent call last):
AttributeError: ‘myClass' object has no attribute ‘new_attr'>>>Read only attributes?
Another one is that, if there is some kind of collision between the slot and a class attribute, then the class attribute will overwrite the slot and, as there is no __dict__, the class attribute will be read-only.
However if you want to have a __dict__, you can always insert into the __slots__ the ‘__dict__' value, and all these little side effects will go away
But what if I wanted to add the ‘__dict__' value into __slots__ at runtime?
sorry dude but, no can do.
reference: http://mypythonnotes.wordpress.com/2008/09/04/__slots__/:wq
Latest versionReleased:
A multi-armed bandit library for Python
Monty Python Slot Machine App
Project description
A multi-armed bandit library for Python
Slots is intended to be a basic, very easy-to-use multi-armed bandit library for Python.
Author
Roy Keyes -- [email protected]
License: MIT
See LICENSE.txt
Introduction
Monty Python Holy Grail Free
slots is a Python library designed to allow the user to explore and use simple multi-armed bandit (MAB) strategies. The basic concept behind the multi-armed bandit problem is that you are faced with n choices (e.g. slot machines, medicines, or UI/UX designs), each of which results in a 'win' with some unknown probability. Multi-armed bandit strategies are designed to let you quickly determine which choice will yield the highest result over time, while reducing the number of tests (or arm pulls) needed to make this determination. Typically, MAB strategies attempt to strike a balance between 'exploration', testing different arms in order to find the best, and 'exploitation', using the best known choice. There are many variation of this problem, see here for more background.
slots provides a hopefully simple API to allow you to explore, test, and use these strategies. Basic usage looks like this:
Using slots to determine the best of 3 variations on a live website.
Make the first choice randomly, record responses, and input reward 2 was chosen. Run online trial (input most recent result) until test criteria is met.
The response of mab.online_trial()
is a dict of the form:
Where:
- If the criterion is met,
new_trial
=False
. choice
is the current choice of arm to try.best
is the current best estimate of the highest payout arm.
To test strategies on arms with pre-set probabilities:
To inspect the results and compare the estimated win probabilities versus the true win probabilities:
By default, slots uses the epsilon greedy strategy. Besides epsilon greedy, the softmax, upper confidence bound (UCB1), and Bayesian bandit strategies are also implemented.
Regret analysis
A common metric used to evaluate the relative success of a MAB strategy is 'regret'. This reflects that fraction of payouts (wins) that have been lost by using the sequence of pulls versus the currently best known arm. The current regret value can be calculated by calling the mab.regret()
method.
For example, the regret curves for several different MAB strategies can be generated as follows:
API documentation
For documentation on the slots API, see slots-docs.md.
Todo list
- More MAB strategies
- Argument to save regret values after each trial in an array.
- TESTS!
Contributing
I welcome contributions, though the pace of development is highly variable. Please file issues and submit pull requests as makes sense.
The current development environment uses:
- pytest >= 5.3 (5.3.2)
- black >= 19.1 (19.10b0)
- mypy = 0.761
Holy Grail Slot Machine
You can pip install these easily by including dev-requirements.txt
.
For mypy config, see mypy.ini
. For black config, see pyproject.toml
.
Release historyRelease notifications | RSS feed
0.4.0
0.3.1
0.3.0
Monty Python Slots Online
0.2.0
0.1.0
Download files
Monty Python Slot Machine App
Download the file for your platform. If you're not sure which to choose, learn more about installing packages.
Filename, size | File type | Python version | Upload date | Hashes |
---|---|---|---|---|
Filename, size slots-0.4.0-py3-none-any.whl (8.7 kB) | File type Wheel | Python version py3 | Upload date | Hashes |
Filename, size slots-0.4.0.tar.gz (174.8 kB) | File type Source | Python version None | Upload date | Hashes |
Hashes for slots-0.4.0-py3-none-any.whl
Algorithm | Hash digest |
---|---|
SHA256 | 2bc3e51a6223ae3984476f5d1bb272e70ca6d74fbd298fe48c7283cc1c358cc7 |
MD5 | 1ec6d4398f2a36036f452a9c77d0d43c |
BLAKE2-256 | 61dacfca624262fdcce7c043b5e96f06a64019d4a6581a31d5e34ee52b9d30cd |
Slot Machine In Python
Hashes for slots-0.4.0.tar.gz
Python Slots Class Attribute
Algorithm | Hash digest |
---|---|
SHA256 | b02b7b60084b6fe2b0297a14493289c4f7ee88ae374ec518237ca6b13d39e7ae |
MD5 | f91161c95cf32357b2b23b01ca4041c9 |
BLAKE2-256 | 443e20fea85cf3b7054cd90da868b50640e9dc532509567462e623ea1aeefb42 |